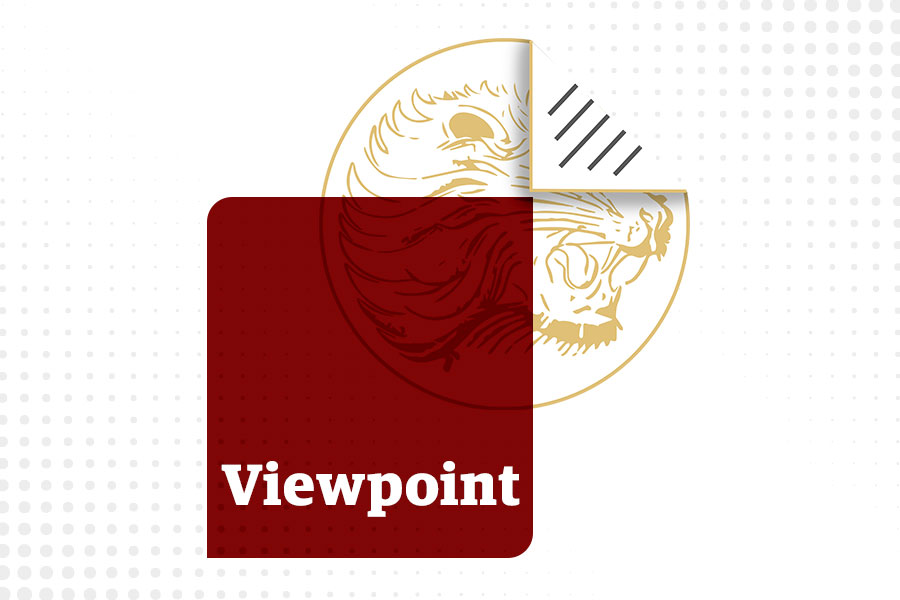
Viewpoints | Jul 27,2024
May 13 , 2023
By Ricardo Hausmann
Deep neural networks (DNNs) have outperformed all other artificial intelligence (AI) technologies, driving significant advances in computer vision, speech recognition, and translation. The emergence of generative AI chatbots like ChatGPT continues this trend, observed Ricardo Hausmann, a professor at Harvard University's John F. Kennedy School of Government and Director of the Harvard Growth Lab, in this commentary provided by Project Syndicate (PS).
I do not intend to talk about how ChatGPT responds when prompted about economic development strategies. It regurgitates reasonable but mediocre ideas seen in its training set. But ChatGPT's design, which has given it far greater capabilities than its creators anticipated, offers a valuable lesson for tackling the complexities of economic development.
For over a decade, deep neural networks (DNNs) have outperformed all other artificial intelligence (AI) technologies, driving significant advances in computer vision, speech recognition, and translation. The emergence of generative AI chatbots like ChatGPT continues this trend.
To learn, AI algorithms require training, which can be achieved through two main approaches: supervised and unsupervised learning. In supervised learning, humans provide the computer with a set of labelled pictures such as "dog," "cat," "hamburger," "car," and so on. The algorithm is then tested to see how well it predicts the labels associated with images it has not yet seen.
The problem with the supervised approach is that it requires humans to go through the tedious process of manually labelling every picture.
By contrast, unsupervised learning does not rely on labelled data. But the absence of labels raises the question of what the algorithm is supposed to learn. To address this, ChatGPT trains the algorithm to predict the next word of the text that is used to train it.
Predicting the next word may seem trivial, akin to the auto-complete function in Google Search. But ChatGPT's model allows it to perform highly complicated tasks, such as passing the bar exam with a better score than most high-performing law students.
The key to such feats lies in the impressive power of this simple learning process. To predict the next word, the algorithm is forced to develop a nuanced understanding of context, grammar, syntax, style, and much more. The level of sophistication it achieved surprised everybody, including its designers. DNNs proved capable of functioning much better without trying to incorporate the theories that linguists had developed into learning language models for decades.
The lesson for economic development is that policymakers should focus on a task that may seem mundane, provided that they would indirectly be forced to learn much more intricate development challenges to excel at it.
By contrast, the prevailing approach in the field of development economics has been to distinguish between proximate causes and deeper determinants of growth and focus on the latter. This approach is analogous to saying, "Instead of trying to predict the next word, understand the context and meaning of the entire book."
In their 2012 book "Why Nations Fail", Daron Acemoglu and James A. Robinson argue that institutions, by affecting the structure of incentives in society, are the ultimate determinant of economic outcomes.
Brown University economist Oded Galor has taken a different approach, emphasizing the complex demographic and technological transformations that brought humanity out of the Malthusian equilibrium and led to longer life expectancy, lower fertility rates, and higher investment in education. Together, these trends boosted women's participation in the labour force and increased the availability of skills needed to sustain technology adoption and economic growth.
But do these theories match the facts?
Over the past four decades, the developing world has indeed undergone many of the radical transformations that Galor described. As the late physician Hans Rosling observed, the gaps between developing and developed countries in life expectancy, infant mortality, fertility, education, university enrollment, female labour-force participation, and urbanization have all narrowed sharply. Reasoning à la Acemoglu and Robinson, developing countries' institutions could not be all that bad if they could deliver progress on so many fronts. In Galor's framework, progress on all these fronts should explain why developing countries caught up so much with the developed world in terms of income.
Except that they did not: the median country is no closer to US income levels than four decades ago.
How is it possible that the narrowing gaps in education, health, urbanization, and female empowerment also failed to narrow the income gap? Why has progress not in the supposed deeper determinants delivered the goods?
Economists invoke a widening technological gap to make sense of this puzzling outcome. More than an explanation, this is a mathematical necessity: if more inputs do not generate more output, something must be making inputs less effective.
To explain this unexpected outcome, it is helpful to note that the few countries that did manage to catch up share two distinctive features: their exports grew much faster than their GDP, and they diversified their exports by shifting toward more complex goods.
To achieve this feat, these successful countries must have adopted and adapted better technologies, adjusted the provision of public goods and their institutions to support emerging industries, and reduced inefficiencies and costs by increasing productivity and training workers. In that process, they may have fixed a bunch of other problems.
A ChatGPT-inspired development strategy would focus on a simple goal: to improve the competitiveness, diversity, and complexity of exports. Figuring out how to do this would force policymakers to learn how to do essential things, just like predicting the next word enabled ChatGPT to learn context, grammar, syntax, and style.
Like early AI programmers who were sidetracked by linguists and their convoluted theories, policymakers have been distracted by too many objectives, such as the 17 United Nations Sustainable Development Goals (SDGs). But applying the ChatGPT approach to economic development could simplify things: just as the language model tries to predict only the next word, policymakers could try to focus on facilitating the next export, as successful countries seem to have done.
While this may seem like a small step, it could lead to surprisingly significant results.
PUBLISHED ON
May 13,2023 [ VOL
24 , NO
1202]
Viewpoints | Jul 27,2024
Radar | Jan 01,2023
Viewpoints | Jul 29,2023
Commentaries | Dec 30,2023
Radar | Apr 08,2023
My Opinion | Mar 01,2024
Commentaries | May 18,2024
Viewpoints | Jun 29,2024
Viewpoints | Aug 05,2023
Fortune News | Mar 09,2019
My Opinion | 113383 Views | Aug 14,2021
My Opinion | 109579 Views | Aug 21,2021
My Opinion | 108592 Views | Sep 10,2021
My Opinion | 106384 Views | Aug 07,2021
Aug 18 , 2024 . By AKSAH ITALO
Although predictable Yonas Zerihun's job in the ride-hailing service is not immune to...
Jul 13 , 2024 . By AKSAH ITALO
Investors who rely on tractors, trucks, and field vehicles for commuting, transportin...
Jul 13 , 2024 . By MUNIR SHEMSU
The cracks in Ethiopia's higher education system were laid bare during a synthesis re...
Jul 13 , 2024 . By AKSAH ITALO
Construction authorities have unveiled a price adjustment implementation manual for s...
Nov 2 , 2024
Addis Abeba, fondly dubbed a 'New Flower,' is wilting under the weight of unchecked u...
Oct 26 , 2024
When flames devoured parts of Mercato, residents watched helplessly as decades of toi...
Oct 20 , 2024
Central Bank authorities have unveiled no less than six new guidelines to fine-tune t...
Oct 12 , 2024
In his inaugural address on October 27, 2024, Taye Atseqesellasie, the fifth presiden...
Nov 3 , 2024 . By HAWI LEGESSE
Ethiopian Airlines Group has extended an invitation to financial institutions and new...
Nov 3 , 2024 . By BEZAWIT HULUAGER
Federal authorities' efforts to secure sufficient fertiliser for the vast agricultura...
Nov 3 , 2024 . By AKSAH ITALO
Parliament received a bill last week mandating year-long training and biennial licens...
Nov 3 , 2024 . By AKSAH ITALO
The federal government is moving to reform the franco valuta scheme after it opened u...